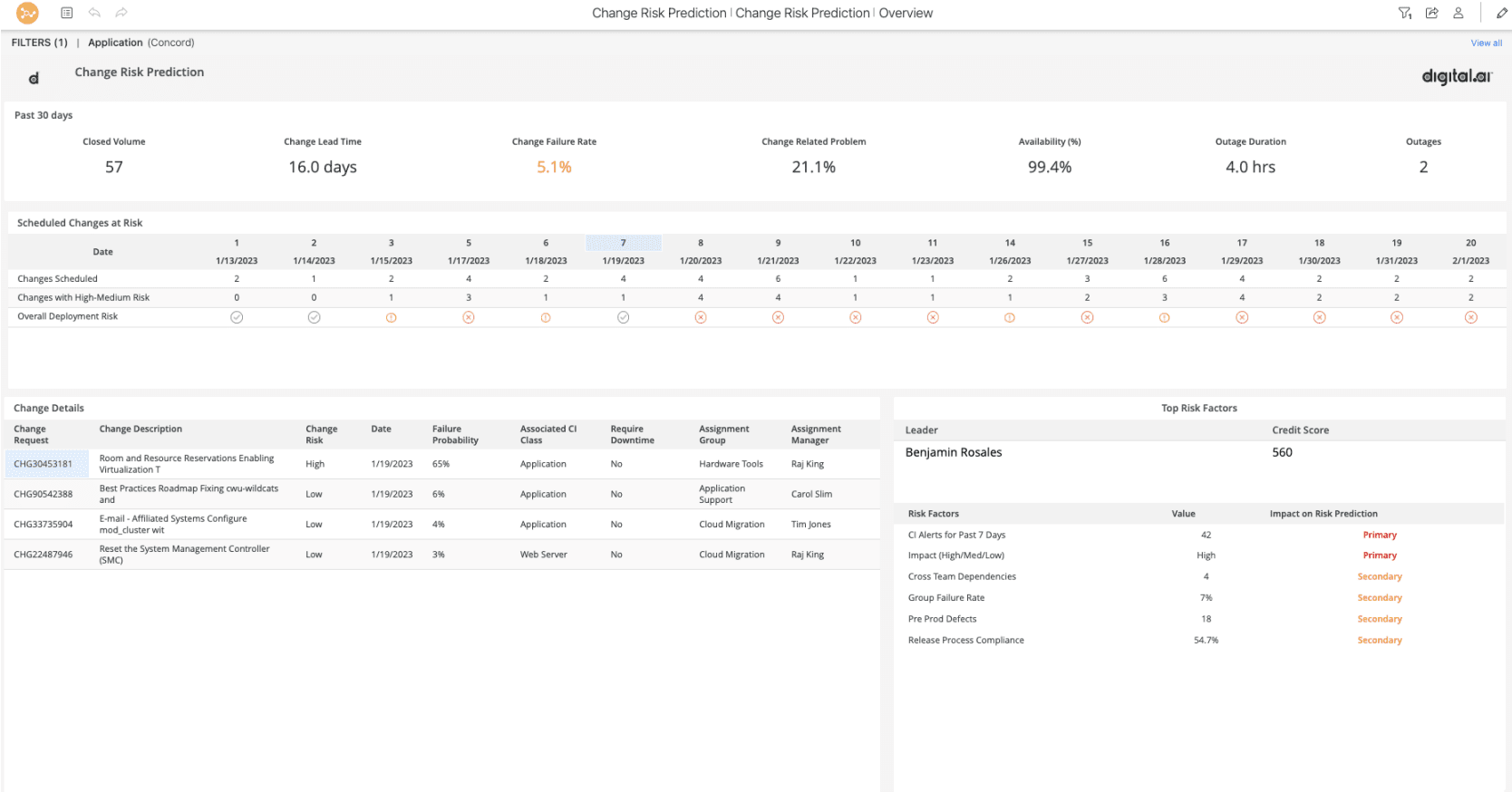
Predictive Intelligence: Change Risk Prediction
Predict potential software change failures and proactively mitigate risks to protect user experience and bolster business outcomes.
AI-Driven Change Risk Impact Analysis
Change Risk Prediction (CRP) is an enterprise-grade AI-powered analytics product designed to help you predict which changes are prone to failure, allowing you to make data-driven decisions to avoid downtime and improve user experience.
With CRP, you can analyze the overall impact of change in applications and businesses, its past and persistent trends in incidents, problems, and outages, the overall change in credit and debit scores for the implemented changes, and the availability of applications. Additionally, the solution incorporates built-in AI/ML models that effectively reduce change failures and associated incident mean time to resolution (MTTR).
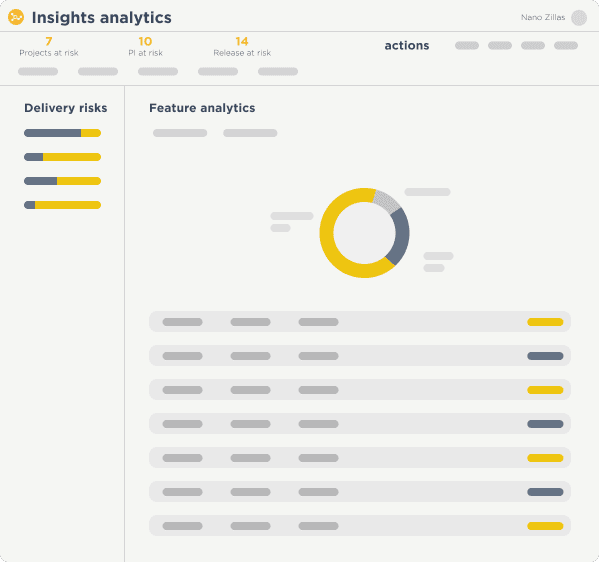
Deliver Software Reliably with AI-powered Analytics
Capabilities
Adopt an AI-driven approach to change success
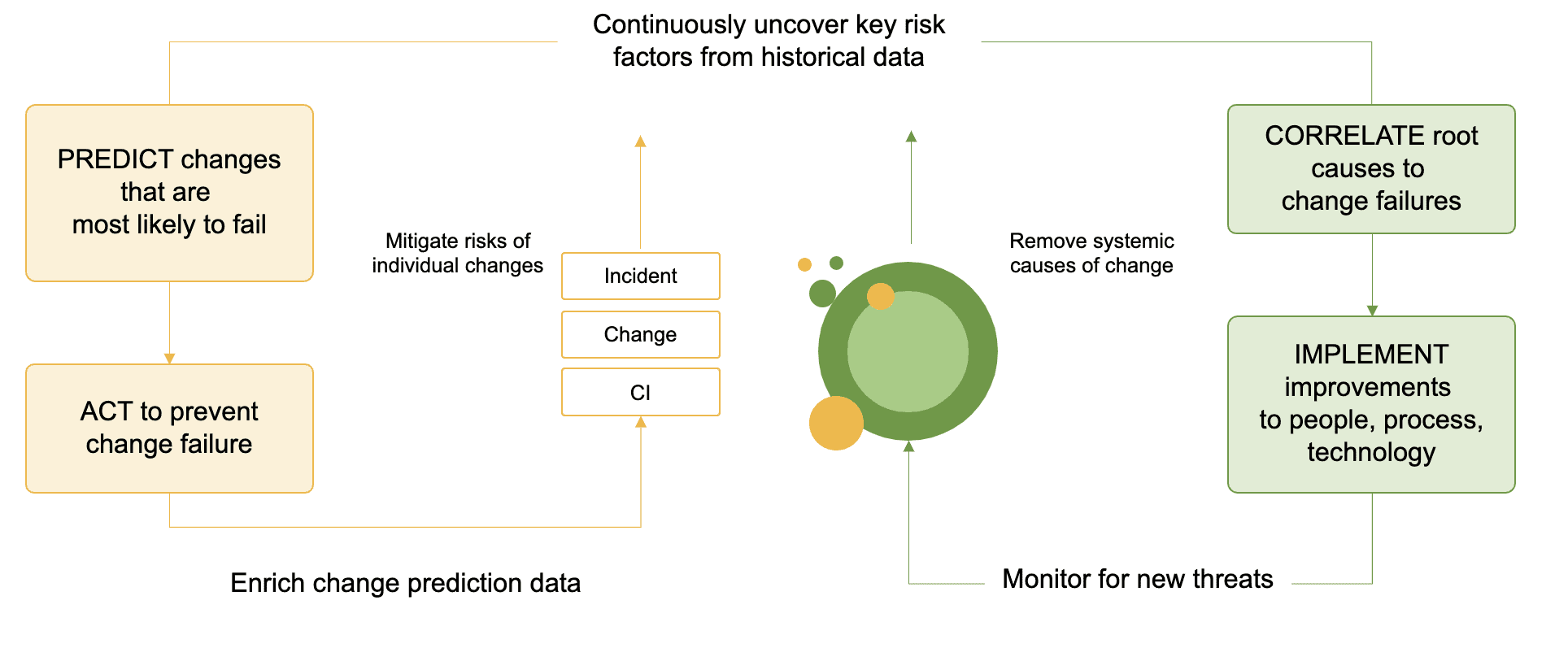

“When information flows easily, things get done. It increases software delivery performance and operational performance.”
Accelerate The State of DevOps Report by Google DORA Community, sponsored by Digital.ai
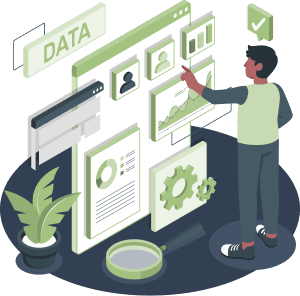
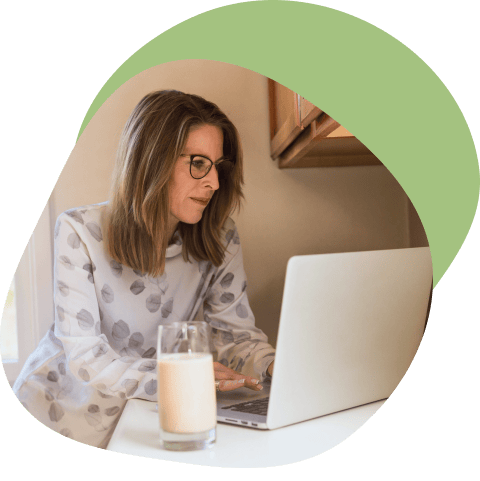
See Digital.ai Change Risk Prediction in Action
We have helped thousands of teams across industries predict, analyze, and make data-driven decisions with Digital.ai Intelligence. Contact us to learn more.